In sync with the world bouncing back from the recent global crisis, I'm beginning to pick up the slack on two years spent waiting for everything to improve, and this is one of the projects I thoroughly enjoyed sinking my teeth into.
Two years ago, in early 2020, I participated in the Political Data Science course run at the Bavarian School of Public Policy by Orestis Papakyriakopoulos (who has since gone on to Princeton), and found myself in a group project scenario. Thankfully, this one paired me up with Linlin Chen, a stunningly clever colleague whom I'm grateful to now call a friend.
Together we looked at the hot topic of the hour — COVID-19 lockdown policies — and on the example of US states, which gave us plenty of easily accessible and comparable data, we scrutinized their causes. You can find our analyses and conclusions in a nifty, interactive(!) presentation embedded above and stored here. Our (fairly simple) R code is also up on GitHub.
The dataset we used contained a variables we could collect from publicly available sources, including the following:
- CoronaNet Research Project (policy data);
- Data Repository by the CSSE at Johns Hopkins University (daily COVID-19 deaths per US state);
- US Bureau of Labor Statistics (new weekly unemployment claims);
- US Bureau of Economic Analysis (GDP per capita for each US state); and
- US Census Bureau (regionality of US states).
Using a panel data regression, we investigated the obfuscated interdependencies of these variables within their multidimensional set.
Some of the interesting results we found were that, on average, Democrats acted to enforce lockdown policies four days before Republicans, the GDP per capita of a state was not a statistically significant factor in this decision, and (all things being equal) California was the most trigger happy of the US states, while South Dakota was the least.
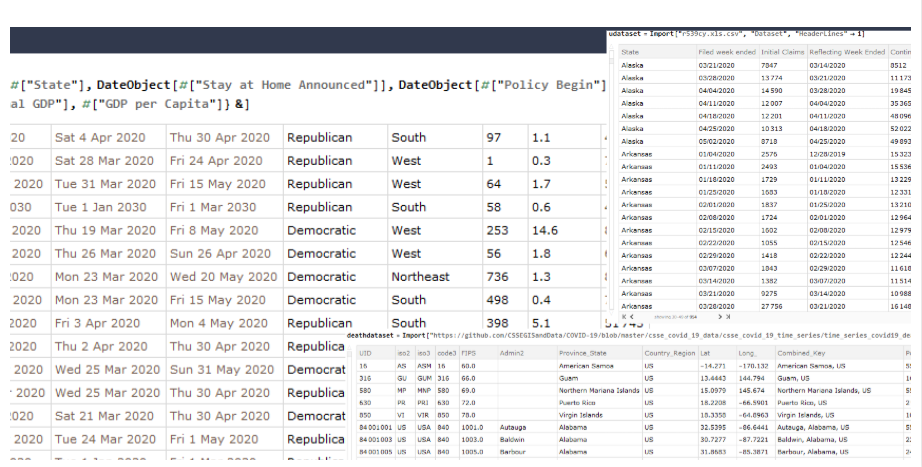
Of course, this analysis wasn't perfect, and neither was our dataset. The differing ways in which different US states tracked (and reported) COVID-19 deaths or new unemployment claims certainly affected our results, and the varying strengths of their lockdown policies (as well as their enforcement by individual counties) probably weren't comparable, but few things in policymaking can be called straightforward or easy.
In its very essence, policymaking is interdisciplinary and the best data analyses can do here is give us complex answers to complex questions. Strict lockdown policies were not always the best call — carrying with them the unintended consequences of more frequent instances of domestic violence or dire straits for entire economic sectors — and the tricky balance between enacting and enforcing a policy cannot be overlooked, either.
Knowing that future societal and political responses to COVID-19 safety measures were affected by those first, rushed acts of lockdown, looking at the data and better understanding the interplay between these major factors can help us take a step toward looking beyond the obvious.